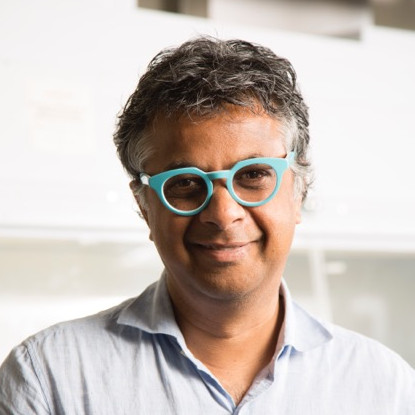
Columbia University
New York, NY
Polymeric membranes, which present an efficient solution for emergent technologies, such as CO2 capture and natural gas purification, are effective at the selective and efficient transport of gases. The field of polymer membrane design is primarily based on empirical observation, which limits the discovery of new, advanced materials most appropriate for separating a given gas pair. Instead of relying on exhaustive experimental investigations, we apply machine learning on a limited set of experimental gas permeability data for six different gases in ~700 polymeric constructs that have been measured to date to predict the behavior of over 10,000 homopolymer architectures that are currently known. This machine learning technique, which only uses a small body of experimental data (and no simulation data) to accurately predict the behavior of large classes of polymers, evidently represents a novel means of exploring the vast phase space available for polymer membrane design in a manner that is orders of magnitude more efficient than typical empirical observations. However this approach suggests that the current polymers used in membrane applications represent the best that this template can provide – thus new platforms are required to improve performance. We thus propose novel membranes based on polymers mixed with nanoparticles (GNPs). The issues raised by these methods and ways to use and leverage them to systematically improve performance is the second major focus of my tutorial.
SHORT-BIOUndergrad education BTEch, Chem Eng., IIT Madras; SM and ScD, MIT, Chemical Engineering, 1987; Post-doc, IBM; Penn State 1989-2002; Rensselaer Polytechnic Institute, 2002-2006; Columbia 2006-; Brookhaven National Labs 2021-; IIT Madras Chevron Visiting Prof, 2018-; IIT Mumbai, Distinguished Visiting Prof., 2022-; Editor Soft Matter; Department chair, Chem Eng., Columbia 2010-2016; Polymer Physics Prize, APS, 2022.